Machine Translation for Low-resource Finno-Ugric Languages
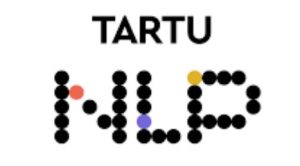
Scientific partners involved
The University of Tartu is the leading university in the Baltics in the field of information technology and is one of the 250 best universities in the world in the ranking of Times Higher Education Universities in the field of Computer Science. Examples of the University’s Chair of Natural Language Processing’s research directions include machine learning and data mining on text data, machine translation from one natural language into another, summarization of a long text document, automatic analysis of grammatical correctness, word meanings and sentence structure.
Technical/scientific challenge
Neural networks have caused rapid growth in output quality for many natural language processing tasks, including neural machine translation (NMT). However, the output quality crucially depends on the availability of large amounts of parallel and monolingual data for the covered languages. Due to lack of training material, several lower-resource Finno-Ugric languages are not included in the existing massively multilingual models. In terms of the number of speakers, they range from 20 near-native speakers of Livonian to several hundred thousand speakers of Mordvinic languages.
Solution
An NMT system was developed between 20 low-resource Finno-Ugric languages and 7 high-resource languages. Monolingual corpora was collected mainly by crawling texts off the web and combining with pre-existing corpora. Three main categories of texts can be distinguished: news, Wikipedia, and biblical. All the NMT systems were trained on the LUMI supercomputer. All models were fine-tuned with the Fairseq framework implementation of M2M-100 for 350k updates with a batch size of 3840 tokens (the number was chosen to match earlier versions of models trained with the Huggingface implementation of M2M-100). The models were fine-tuned on 4 AMD Mi250X GPU-s.
Scientific impact
More that 20 Finnish-Ugric languages can now be translated using the machine translation engine of the University of Tartu. Most of these languages were added to a public translation engine for the first time. The translation engine allows researchers to translate materials that would otherwise be incomprehensible to them. It provides an opportunity to better study the history of languages and regions without knowing the respective language. Moreover, since most of Finno-Ugric languages are not widely spoken today, a translation engine is necessary to preserve these languages.
Benefits
- Collection of parallel and monolingual corpora that can be used for training NMT systems for 20 low-resource Finno-Ugric languages
- Expansion of the 200-language translation benchmark FLORES-200 with manual translations into nine new languages (Komi, Udmurt, Hill and Meadow Mari, Erzya, Livonian, Mansi, Moksha and Livvi Karelian)
- The collected data can be used to create NMT systems for the included languages and investigate the impact of back-translation data on the NMT performance for low-resource languages
Cosmic Ray-based Solutions for 3D Imaging
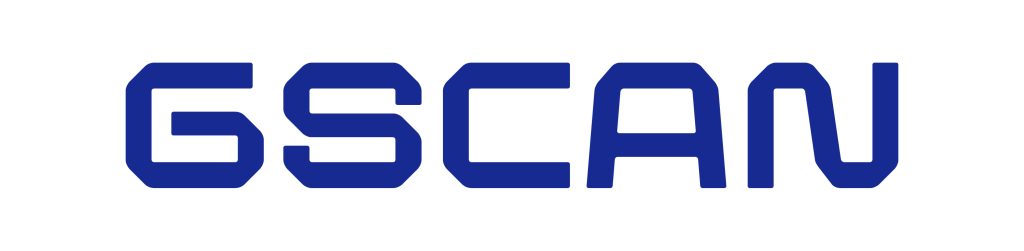
Industrial organisations involved
GScan was founded in 2018 to revolutionise inspection, security and medical scanning markets using Muon Flux Technology (MFT). GScan, as the pioneer of MFT having unique IP, tech & sales know-how in the field, is developing a new generation of Non-Destructive Testing (NDT) scanners and tomography systems for infrastructure management applications.
Technical/scientific challenge
To keep the surrounding environment safe and ensure its longevity, careful assessment, maintenance and investment plans are required. However, currently there is no efficient way of obtaining the information required for more efficient use of assets and reducing risks for critical infrastructure.
Solution
Capitalising on the power of natural cosmic ray tomography, the technology tracks the trajectory changes or absorption of particles (muons, electrons, positrons) as they pass through the object of interest, thereby extracting crucial statistics about its material and shape. These insights are then translated into 2D and 3D visualisations of both internal and external geometries, along with data on chemical composition. The comprehensive output we deliver provides in-depth insights into the objects and materials under scrutiny – all meticulously tailored to fulfil our customers’ unique requirements. HPC plays an important role in translating the collected data into visualisations.
Business impact
With time and space related digital data in terabytes, the detailed process of reconstruction enables us to see inside of structures what was not possible before.
Benefits
- With HPC we can process the data and do our reconstructions faster
- With faster reconstruction it is possible to apply wider range of algorithms during the post processing
- With a wider range of algorithms the capability and efficiency of the technology grows and with better muon flux technology the world can become safer thanks to more reliable data about critical infrastructure
Machine Translation Post-Editing
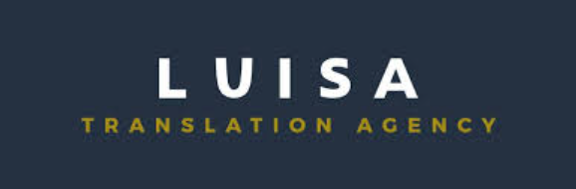
Industrial organisations involved
Luisa Tõlkebüroo OÜ is the biggest translation agency in Estonia. The company offers more than 50 services – including sworn translation, simultaneous and consecutive interpretation, layout work, machine translation and post-editing, subtitling and localisation.
Technical/scientific challenge
The company needed a custom-made machine translation system to reduce the time of translations. As the company had no previous experience neither in natural language processing nor in machine learning, they collaborated with the TartuNLP team.
Solution
Training of the machine translation model was conducted by using University of Tartu HPC centre’s Rocket cluster. The company needed a custom-made machine translation system to reduce the time of translations. As the company had no previous experience neither in natural language processing nor in machine learning, they collaborated with the TartuNLP team. Training of the machine translation model was conducted by using University of Tartu HPC centre’s Rocket cluster.
Business impact
Thanks to rapid advances in the technology and extensive translation memory, the company is able to offer machine translations with post-editing in a range of language combinations and on a range of topics.
Benefits
- The innovative translation tool helps to save valuable time and human resources
- Creation of high quality reference dataset for future developments.
- Innovative application of deep learning techniques in cloud masking.
An accurate AI-based Cloud Mask Processor for Sentinel-2

Industrial organisations involved
KappaZeta is a science-driven remote sensing company aiming to make space a valuable asset for everyone. KappaZeta’s expertise is in using SAR (radar) satellite data, incorporating it with optical satellite data and providing some of the most accurate AI models on the market. The key area of focus is agriculture.
Technical/scientific challenge
Cloud masking is an essential step for the pre-processing of optical satellite imagery. KappaZeta addresses the problem by introducing KappaMask, an AI-based cloud and cloud shadow masking processor for Sentinel-2, which carries an optical instrument payload that samples 13 spectral bands. As a cloud detector, KappaMask uses a large convolutional segmentation model. Faster model convergence during training can be achieved by using larger batch sizes of the training data, which means more GPU memory is needed. Additionally, faster CPUs are required for shorter data loading times to increase the training speed even further.
Solution
KappaMask was trained on an open-source dataset and fine-tuned on a Northern European terrestrial dataset which was labelled manually using the active learning methodology. The training was performed on the University of Tartu’s HPC Centres’ high-performance compute nodes. Powerful GPUs and CPUs were applied to substantially speed up the training of the model.
Business impact
KappaMask is an open source project. All the results, final software and source code will be freely and openly distributed in GitHub. Openness and accessibility of the software should directly translate into greater usage.
Benefits
- Reliable cloud mask processor for Northern Europe region, which is compatible with ESA Sentinel-2 L2 processing chain.
- Creation of high quality reference dataset for future developments.
- Innovative application of deep learning techniques in cloud masking.
Self-driving technology for a Level 4 autonomous car
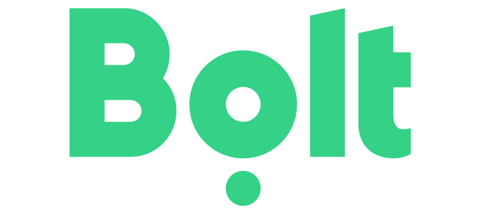
Industrial organisations involved
Bolt is an Estonian mobility company that offers vehicle for hire, micromobility, car-sharing, and food delivery services headquartered in Tallinn and operating in over 400 cities in over 45 countries. In partnership with the University of Tartu, the company developes self-driving technology for a Level 4 autonomous car.
Technical/scientific challenge
Autonomous cars acquire up to 357 GB/hour of data during test drives. Autonomous car engineers needed a system to store and easily access those test logs.
Solution
Acquired test logs are copied to HPC storage, into appropriately guarded directory. Regularly cron job processes those log files into metadata stored in MongoDB database. Processing is distributed over cluster and happens in parallel. Longest logs can take up to 24 hours to process, so processing them sequentially would be very time-consuming. On top of MongoDB sits custom-made application that allows filtering of test sessions and browsing them using Webviz visualization tool. Visualization tool accesses the raw sensor data from HPC storage.
Business impact
With the growing demand for ride-hailing services, autonomous vehicle technology will provide a solution for transportation problems on an increasingly broader scale.
Benefits
- Custom database application and visualization tool enables easy analysis of the logs
- Thanks to distributed processing in the cluster the metadata about the drives usually shows up already next morning
- Thanks to petabytes of storage at the HPC Centre, the company can keep all the data they need
Large collections of European HPC success stories are available on the FF4EuroHPC and EuroCC webpages.